AI Execution Playbook
Why AI Execution Matters—and Why New Dialogue AI Wins
Date: March 26, 2025
AI can transform your business—or waste your time. Execution is the difference. This playbook from New Dialogue AI (www.newdialogue.com) shows why most fail and how we deliver: fast, secure, and proven.
AI - Real Gains or Real Losses?
When AI is done right, it delivers:

But most companies never get there.


- The customer used New Dialogue AI chatbots to deliver public-facing emergency services.
- 600+ real queries answered³ during Pilot — from safety procedures to real-time emergency services requests
- Productivity savings of up to $2.63M/year⁶, based on time recovered from faster answers and document automation.

- Sources: ResearchGate (2024):
Average internal search time per employee
ABS (Australian Bureau of Statistics), 2024: - New Dialogue AI internal modeling (2025)6
How New Dialogue AI Fixes Four Common Pitfalls
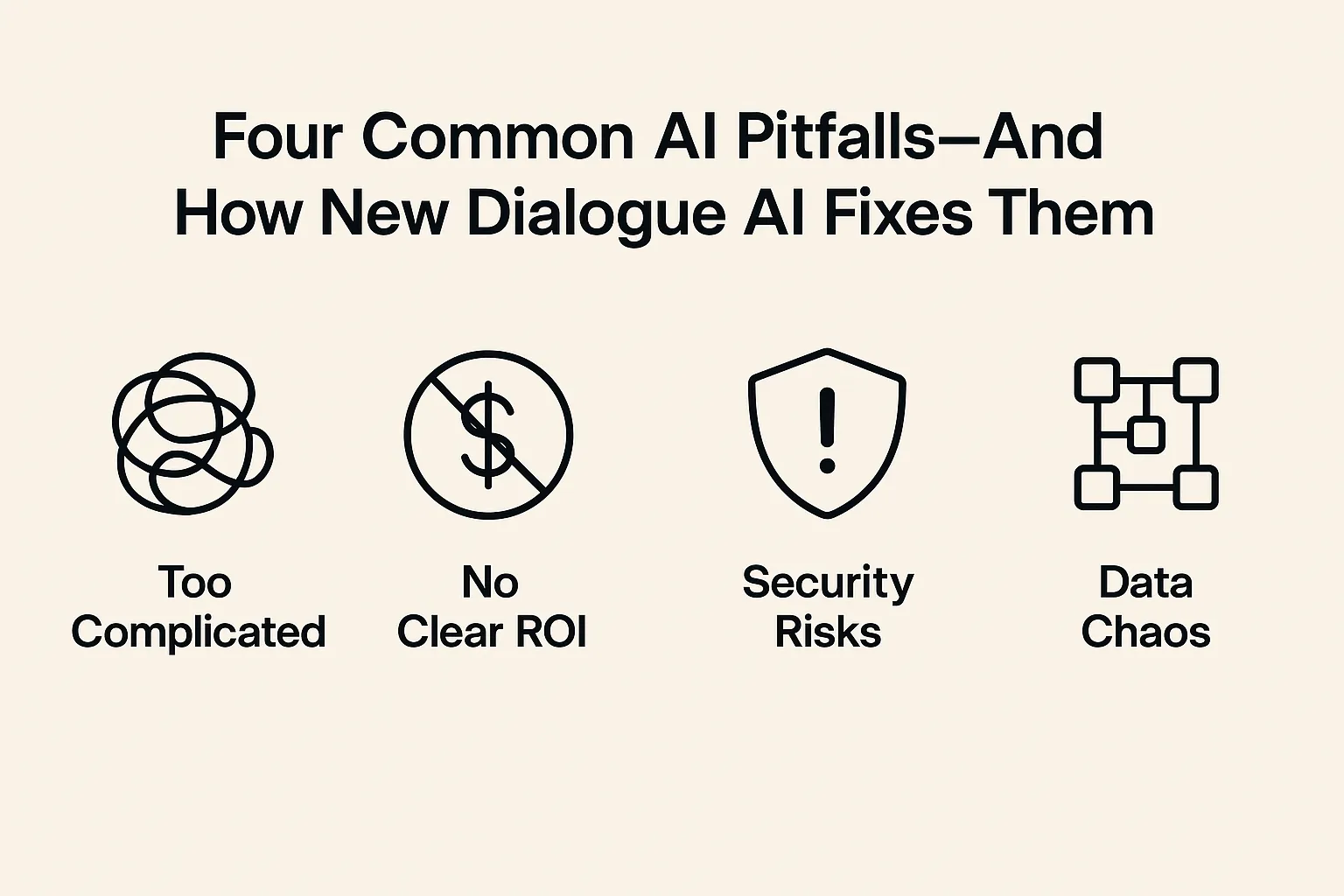
1. Too Complicated
- Problem: Roughly 70% of GenAI projects are still stuck in pilot, unable to scale.7
- Fix: New Dialogue AI deploys in weeks, not months.
- Action: Day 1—Instruct IT, “Deploy AI solutions in weeks, not months.”
2. No Clear ROI
- Problem: AI without clear productivity benefits is ignored by leadership.
- Fix: New Dialogue AI immediately demonstrates quantifiable productivity savings.
- Action: Day 1—List your key productivity drains (e.g., inefficient internal searches, delayed client responses) and target them clearly.
3. Security Risks
- Problem: 8.5% of prompts in public GenAI tools leak company data.8
- Fix: New Dialogue AI’s secure, private Retrieval-Augmented Generation (RAG) ensures compliance and data security.
- Action: Day 1—Immediately ban use of public AI in favor of secure, private AI.
4. Data Chaos
- Problem: 63% of organizations lack or are unsure if they have the right data management practices for AI.9
- Fix: New Dialogue AI connects directly to your existing documents and data using Retrieval-Augmented Generation (RAG) — no migrations or reformatting required.
- Action: Day 1 — Choose one high-impact use case (e.g. onboarding, approvals, incident response). Identify the 5–10 documents or data sources it depends on, and ensure they’re accurate, current, and accessible.
Understanding Retrieval-Augmented Generation (RAG)
Why RAG Matters
Retrieval-Augmented Generation (RAG) is what happens when your AI stops hallucinating, and starts acting like it passed the background check.
In plain terms, RAG combines the power of large language models (LLMs) with real-time access to your company’s own knowledge — think ChatGPT, but with a corporate memory.
Instead of relying solely on what the AI was trained on (which may be outdated, irrelevant, or wildly confident - but wrong), RAG allows the model to retrieve relevant documents, data, or content from your own systems — manuals, contracts, CRM entries, spreadsheets, even that one PDF from 2017 no one wanted to read — and augment its response with that context.
The result? Fewer hallucinations, more accurate answers, and your AI actually sounding like it works here.
Why should you care?
Because RAG bridges the gap between generic AI and your business intelligence. It powers internal tools like smart chatbots, field support assistants, and sales copilots that don’t just guess—they know. Your teams get instant, context-aware answers, and your IT team doesn’t get 17 more integration requests.
RAG solves this issue by retrieving and referencing accurate, relevant, real-time data directly from your organization's trusted sources; file repositories, Intranet, Client Portal, etc.
Benefits of RAG
- Accurate, Context-Aware Answers:
RAG pulls in real-time, domain-specific information from your own data sources, reducing hallucinations and boosting relevance. - Faster Knowledge Access:
Instead of searching through documents, teams get instant, conversational access to internal content — like manuals, policies, or client info. - AI That Actually Understands Your Business:
By grounding responses in your content, RAG turns generic LLMs into specialized assistants tailored to your workflows and language.
How RAG Impacts AI Deployment
- Private RAG LLMs are ideal if you want control, security, and company-specific intelligence baked into your AI.
- Public AI models are great for general-purpose use, early prototyping, or quick experimentation — but lack business context and data control.
RAG improves AI’s ability to generate fact-based, relevant answers, by retrieving trusted information before responding.

How RAG Impacts AI Deployment
- Public AI (e.g., ChatGPT, Bard) – Generates responses based on pre-trained datasets, which may not be up-to-date or specific for discrete business use. Public AIs do not have direct access to private company data, which can lead to generic or unreliable responses for operational-type queries being used by staff.
- Private AI (New Dialogue AI) – Uses internal data sources with RAG, retrieving trusted business documents, reports, and policies before generating a response. This ensures fact-based, context-aware, and secure answers aligned with company knowledge.
Key Takeaway
What Makes New Dialogue AI Different?
New Dialogue AI is a powerful, purpose-built solution for implementing Retrieval-Augmented Generation (RAG) with your own internal knowledge. It enables organizations to create intelligent, conversational AI experiences that are grounded in real, up-to-date business content — not generic internet data.
With New Dialogue AI, you can connect your chatbot to your company’s private knowledge base — whether that’s PDFs, intranet pages, SOPs, product guides, or CRM data. Instead of hallucinating or offering vague responses, the AI retrieves and references relevant documents behind the scenes, delivering accurate, context-rich answers to employees or customers instantly.
Setup is fast, no code, and doesn't require IT involvement. You simply upload your content or link to your knowledge sources, and the AI is ready to chat — securely and privately.
Whether you're supporting a field team that needs instant access to site instructions or enabling your sales reps to query CRM data with natural language, New Dialogue AI scales with you. It makes knowledge accessible through a familiar, intuitive chat interface — across web, mobile, or internal tools like Slack.
In short, New Dialogue AI gives you a smarter, faster, more secure way to activate RAG, and puts your operational intelligence into everyone’s hands — right when they need it.
How We Reduce Risk
Following are some key points on how using New Dialogue AI with your internal knowledge reduces risk:
- Eliminates Data Leakage to Public AI Models
New Dialogue AI operates securely with your internal data, ensuring sensitive information never leaves your environment, or gets exposed to third-party AI services. - Ensures Accurate, Policy-Compliant Responses
By grounding answers in your vetted internal documentation (e.g., SOPs, HR policies, compliance manuals), the AI reduces the risk of misinformation or off-brand advice. - Provides Auditable, Transparent Knowledge Access
Every AI response is traceable back to a source document, making it easier to review, verify, and ensure accountability — crucial for regulated industries or internal audits.
New Dialogue AI vs. The Rest
Here's a comparison table outlining how New Dialogue AI, Public AI models (like ChatGPT, Gemini, Claude), and Custom Open-Source LLM solutions stack up across key categories for a 500-person company.
This comparison is based on typical characteristics of current AI tools and implementation models. Individual tools may vary depending on setup, usage, and vendor capabilities.
Subscription or usage-based; cost scales with use
High upfront + ongoing maintenance & infra costs
AI Use Cases: Where AI Delivers the Most Value
From RAG to Real Business Impact
Previously we reviewed how Retrieval-Augmented Generation (RAG) ensures AI retrieves accurate, operational-specific knowledge. However, understanding how AI retrieves data is just one piece of the puzzle. The real question is: Where does AI create the most impact in your organization?
Many still view AI as a parlour trick; conjuring photo realistic imagery from descriptions, generating a list of the top five places to visit in Europe, how to calculate the volume of water in the average swimming pool, etc. Leaders moving forward are embedding AI into their operational workflows to unlock trapped knowledge, automate high-value processes, and gain a real competitive advantage.
This section explores how organizations across industries are leveraging a Private AI—not just as an information tool, but as a core driver of efficiency, decision-making, and even forecasting what they might be missing from their core services and products offerings.
RAG shines when customers ask nuanced questions that require pulling info from long docs, manuals, policy pages, or knowledge bases — e.g., “What’s the return process for international customers with warranty extensions?”
Product or Technical Documentation Support
For technical products or SaaS, RAG can answer questions by referencing your live product documentation, guides, or API docs — much faster and more accurately than scripted bots.
Multilingual Self-Service Portals
RAG models can dynamically retrieve answers in the user’s preferred language by referencing translated internal content — reducing the need for duplicating support materials across languages.
RAG isn’t great for situations that require live data access, like “Where’s my order?” or “What’s my current subscription status?” — these need direct system integration, not retrieval.
Account-Specific or Highly Personalized Issues
Anything involving user identity, authentication, or personal account data (password resets, cancellations) should be handled via secure, authenticated channels — not open-ended generative chat.
Emotionally Sensitive or High-Escalation Scenarios
Complaints, refunds, or customer frustration cases may require human tone, empathy, or negotiation — areas where AI still risks sounding cold, generic, or making matters worse.
Sales reps can instantly ask questions like, “What’s the latest messaging against Competitor X?” or “Which case study is best for a healthcare client?” — with the AI pulling directly from internal battlecards, pitch decks, and case libraries.
Deal & Account Research Based on Internal Systems
RAG can be connected to internal notes, CRM entries, and playbooks to answer questions like, “What’s the latest update on the ACME deal?” or “What’s our sales approach for logistics companies?”
Enablement for New RepsInstead of digging through Notion pages or asking their manager 15 questions a day, new hires can ask the AI things like, “What’s our sales process?” or “How do we handle procurement objections?” — pulling directly from internal training content.
Cold outreach or personalized follow-ups benefit from human nuance, creativity, and emotional intelligence — areas where RAG can feel robotic or miss key subtleties (e.g., referencing someone’s LinkedIn post or aligning with their tone).
Sensitive Negotiation or Pricing Strategy Decisions
Complex deal-making often involves context, nuance, tone, and internal judgment. RAG might offer outdated or overly general info, and it's risky to rely on it for pricing moves or discounts without guardrails.
Real-Time CRM Updates or ActionsRAG isn’t built for live updates or transactions like “Update the deal stage” or “Log this call” — that’s better handled with native CRM integrations or automation platforms.
New hires can use RAG-powered chat to ask contextual questions like “Where’s the brand voice guide?” or “How do we handle procurement for new vendors?” — reducing manager dependency and speeding up ramp-up.
Unified Search Across Disconnected Docs & Platforms
RAG can ingest and index knowledge from multiple sources (wikis, PDFs, intranet, Google Drive, SharePoint, etc.) — then provide employees with direct answers instead of making them search through folders and pages.
Operational Support & “How-To” Guidance
Employees can ask questions like “How do I submit an expense report?” or “What’s the process for requesting client access?” — and get precise answers based on current SOPs and HR policies
RAG is only as smart as the content it's fed — if critical knowledge lives in people’s heads or Slack threads, it won’t help unless that info is captured in accessible documents.
Highly Sensitive or Restricted Knowledge
Unless carefully gated, RAG can surface documents or data that shouldn’t be broadly accessible — so it’s risky for orgs with poor content permission hygiene.
Dynamic, Frequently Changing Data
If the information changes daily (e.g., team calendars, project status updates), RAG may serve outdated responses unless regularly re-indexed or connected to live data sources.
Teams can ask operational questions like “What’s the protocol for reporting a security breach?” or “Do we require third-party NDAs for contractors?” — with the AI pulling answers from your compliance manuals or legal docs.
Audit & Evidence Prep Support
During audits, RAG can help quickly locate required documentation, certifications, or logs — saving time and reducing stress during prep and response phases.
Policy & Regulation Search Across Vast Documentation
RAG can instantly surface relevant clauses or references from internal policies, contracts, and external regulations (e.g., GDPR, ISO standards), answering questions like “What’s our data retention policy for EU customers?”
Without robust content permissioning, RAG can accidentally surface restricted or confidential information — risky for compliance-heavy industries like finance, healthcare, or defense.
Making Final Legal or Regulatory Judgments
RAG can support research but shouldn’t replace expert legal review — interpretations of law, contract disputes, or regulatory positions require human legal expertise.
Real-Time Monitoring or Incident Detection
RAG isn’t built for monitoring transactions, logs, or behavior — it's not a replacement for GRC tools, anomaly detection systems, or SIEM platforms.
New hires can use RAG-powered chat to find resources like “Where’s the expense template?” or “How do I get a security badge?” — reducing reliance on managers and speeding up productivity.
Self-Service for Everyday Processes
Employees can resolve things like “What’s the procedure for booking annual leave?” or “How do I access the LMS?” — without needing to raise a ticket or email HR.
Instant Answers to HR & Policy Questions
Employees can ask questions like “How do I request parental leave?” or “What’s our hybrid work policy?” and get answers instantly, pulled from your internal handbooks, HR portals, or policy docs.
Employees may need help with topics like harassment, compensation disputes, or whistleblowing — these are better handled by trained professionals in private, secure channels.
Personalized Coaching or Emotional Support
RAG lacks emotional intelligence — it can’t provide empathetic guidance or respond appropriately to mental health concerns, workplace conflict, or personal issues.
Real-Time Task Execution or Workflow Approvals
RAG doesn’t handle transactional tasks like “Submit my leave request” or “Approve this timesheet” — it’s best used alongside HR systems, not as a replacement for them.
When support reps are handling tickets, RAG can quickly surface relevant knowledge base articles, past resolutions, or internal playbooks to assist responses in real time.
Troubleshooting Guidance from Documentation
RAG can pull precise steps from user manuals, help centers, and internal wikis when users ask things like:
“Why is my device not connecting?” or “How do I reset my password?”
Developer or API Support
For software products, RAG can answer technical questions by retrieving info from API docs, SDK references, or internal engineering wikis — e.g., “What’s the payload for this endpoint?”
In high-stakes scenarios (e.g., outages, security breaches), human judgment, live collaboration, and system access are essential — RAG is too static and generic for crisis response.
Real-Time System Diagnostics
RAG can’t run commands, analyze logs, or check system status — it doesn’t interact with live systems or data.
Personalized Account or System Data
Questions like “Why is my server down?” or “What’s my current usage?” require access to live, user-specific backend data — beyond RAG’s retrieval capabilities.
During proposal cycles, teams can query historical RFP responses, pre-approved language, or compliance requirements — reducing duplicate work and maintaining consistency.
Answering Policy & Process Questions
Teams can ask, “What’s the process for onboarding a new vendor?” or “Where’s the approved supplier list?” — with RAG pulling answers directly from procurement manuals, internal policies, or shared drives.
Vendor & Contract Information Retrieval
Quickly surface data from vendor contracts, SLAs, and compliance docs by asking, “What are the payment terms for Vendor X?” or “When does our contract with Supplier Y expire?”
RAG won’t track delivery status, issue POs, or alert on SLA breaches — those need integrated, real-time procurement tools.
Transactional Procurement Tasks
Tasks like submitting purchase orders, approving vendors, or managing budgets require workflow execution — not just document retrieval.
Sensitive Vendor Negotiations or Pricing Strategy
Contract negotiations, pricing decisions, or risk assessments often involve nuanced judgment and confidentiality — areas where AI-generated content must be carefully reviewed by humans.
AI Readiness Scorecard
How ready are you for AI deployment? Use the following scorecard to determine your organizations’ signal of readiness.
The following is a quick diagnostic to surface where AI is already delivering value, and where your team may need support.
AI-led operations deliver 30–40% efficiency gains — Accenture, 2024
(GenAI helped teams complete tasks 25% faster — MIT Sloan & BCG, 2023)
See KPI’s part 2
Score:
- 5–6 Yes → You're AI-ready. Start scaling with confidence!
- 3–4 Yes → You’ve got momentum—close the gaps to unlock full value.
- 0–2 Yes → Time to reassess. Focus on fast and clear wins to build trust and ROI.
Part 2: Executing an AI strategy with New Dialogue AI
In Part 1, we highlighted the stakes.
In Part 2, we deliver a clear 90-day roadmap to put AI into action; drive measurable productivity gains, plan revenue expansion, and develop a lasting competitive advantage.
Using New Dialogue AI, you’re not simply adding AI. You’ll be embedding a secure and private subject matter expert into your organization.
What Makes New Dialogue AI Different?
Unlike well known and public AI offerings, New Dialogue AI has been designed to be utilised as a private and secure RAG solution, to leverage your internal data—accurately, contextually, and safely. It deploys fast, reduces complexity, and delivers immediate productivity and profitability gains.
Your AI Strategy Will Succeed If You Address These Execution Risks
What Slows AI Adoption?
- Custom AI models sound impressive but often lead to months of development with no impact.
- Winning Move: Start simple, deliver quick wins, and scale from there.
- AI that isn’t tied to measurable business outcomes will never scale.
- Winning Move: Align AI initiatives with clear, revenue-driving or cost-saving goals.
- AI doesn’t automatically follow company policies—it needs strict governance.
- Winning Move: Build security and compliance in before AI causes risk exposure.
The AI Execution Formula—Why Most Fail
85% of AI projects fail to deliver business value. — Gartner
Why? Because most companies rush into AI without a plan for execution.
AI success isn’t about picking the best model—it’s about execution that delivers real impact.
Success Case Example: Companies that embed AI into their core processes see a 40% productivity boost and 20% cost reduction within the first year. — McKinsey
90-Day AI Playbook: From Pilot to Indispensable
The following guide can be used to go from Pilot to Production—solving real problems, driving adoption, and proving value fast. It’s designed to address gaps identified in the AI Readiness Scorecard (e.g., Productivity, Knowledge Access, Adoption).
Days 1–30: Identify a Killer Use Case
Goal
Identify a real, high-impact bottleneck that AI can fix now.
Employees waste 1,072 hours/week searching for internal information (based on internal modelling by New Dialogue using ResearchGate data, 2024)⁶. That’s $55,744/week in lost time—or $2.9M/year for a 500-person company.
What to Do
Deploy New Dialogue AI to solve a real, immediate problem.
✔️ Sales accessing customer data
✔️ Operations finding SOPs
✔️ Marketing pulling campaign insights
✔️ Customer support resolving queries
Skip the long POC.
Run a focused, real-world pilot with measurable outcomes.
Get Data Ready.
Prioritise fast impact over perfect scale.
Define success early, “If this AI disappeared tomorrow, would anyone notice?”
Data Readiness
Map where your critical data lives (e.g., shared drives, CRMs, internal documents). Clean one high-impact dataset (remove duplicates, fix errors, standardize formats). Run a test and assess the quality of AI responses—speed of impact beats size of rollout.
Early Impact
Reclaim 1 hour/week per employee by reducing search time by half (based on reducing 2.14 hrs/week → ~1.07 hrs/week per employee).
New Dialogue AI saves ~4.8 minutes per search—delivering instant answers and adding up to massive gains across your team (AI can deliver up to 40% time savings on high-volume tasks—Accenture, 2024).
Estimated Savings (Based on Modeling)
With a 50% reduction in wasted search time (from 1,072 to 536 hours/week), savings are 536 hours/week = $1.45M/year (for a 500-person company). That’s $1.45M back in your pocket!
KPIs for Success (Days 1–30)
Use these directional KPIs to evaluate your initial AI use case (e.g. AI-powered search or proposal automation). These targets reflect real-world pilots, internal modeling, and practical benchmarks. They are not formal industry standards but are used to guide decision-making and determine whether the pilot is delivering measurable value.
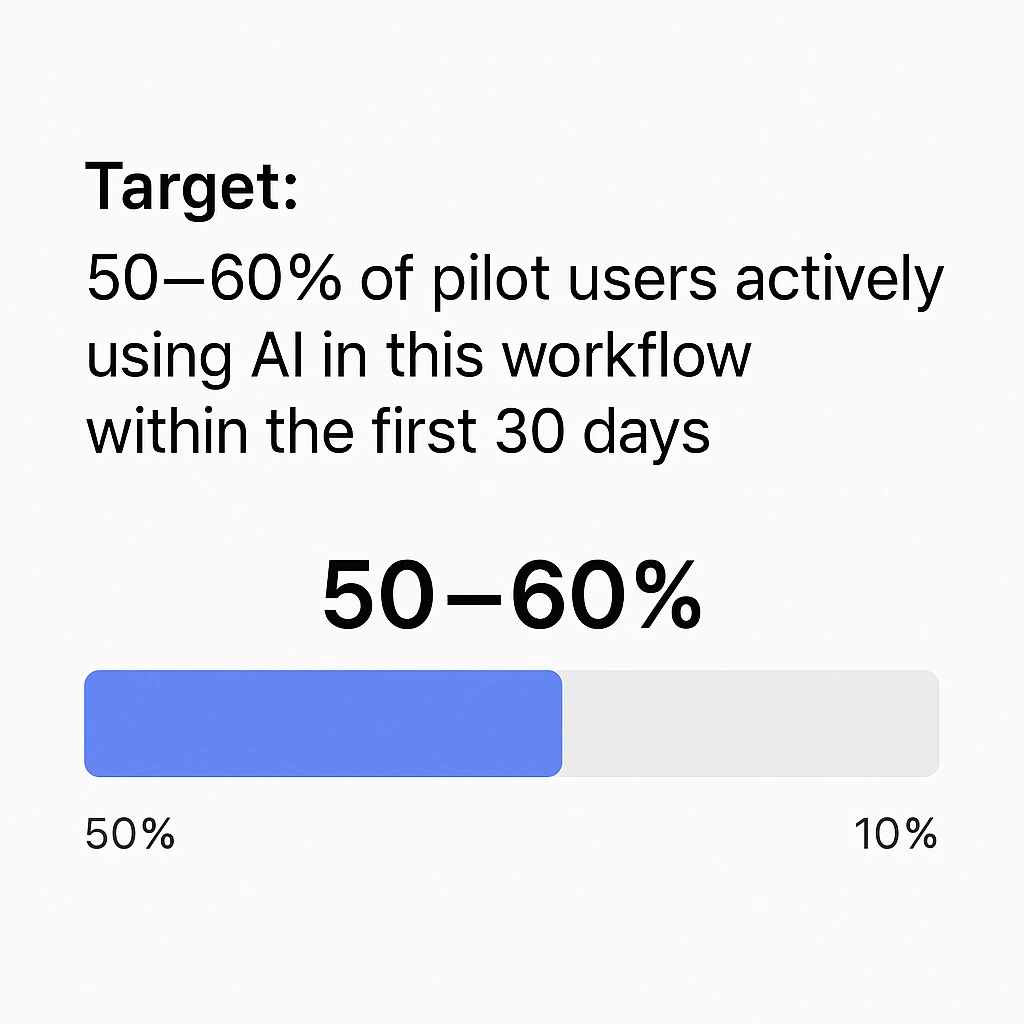
Adoption Rate
Target: 50–60% of pilot users actively using AI in this workflow within the first 30 days
Based on New Dialogue benchmarks from enterprise pilots
Note: No formal industry benchmark available; this is a practical early-stage adoption goal.
Time Saved
Goal: Cut time spent on internal search from 1,072 → 536 hours/week
Based on New Dialogue internal modeling using ResearchGate (knowledge worker search time) ABS wage data


Speed to Answer
Target: AI delivers answers in under 10 seconds for most queries Performance benchmark based on private RAG system design AWS Bedrock: Model Performance
User Sentiment
Prompt: “How much easier is this task now?”
Use in internal surveys to measure perceived ease-of-use and workflow impact.

Challenge to Watch
If adoption is slow, run a 1-hour live demo showing how New Dialogue AI delivers instant answers vs. hunting through folders.
Pitfall to Avoid
Don’t deploy AI on messy data. Poor data = bad answers = broken trust. (Gartner, 2023: Poor data quality is a top cause of AI failure.)
Days 31–60: Integrate AI into Real Workflows
Goal
Make AI part of how work gets done, seamlessly embedded in daily tasks.
AI Integration Actions
- Replace manual search with AI-powered Q&A in real workflows (e.g., customer support reps answering queries, marketing pulling campaign data, operations teams querying sop’s).
- Add AI to common touchpoints (e.g., CRM, email, doc platforms like Slack, Notion, Salesforce).
- Use templates for AI-generated insights (e.g., client summaries, project briefs).
- Gather feedback: Run a quick survey to identify friction points (e.g., “Is AI saving you time?”) and adjust use cases.
Tech Tip
New Dialogue AI integrates with the tools teams already use (e.g., Slack, Salesforce). Test with a small team first to streamline workflows.
Key KPIs
New Dialogue AI integrates with the tools teams already use (e.g., Slack, Salesforce). Test with a small team first to streamline workflows.

Workflow Integration
Target 30%+ of repetitive, high-frequency tasks to be supported or automated by AI.
McKinsey Global Institute (2023) projects that up to 30% of work hours could be automated by 2030, driven largely by AI adoption.
Sources: McKinsey
McKinsey Global Institute (2023)
Generative AI and the Future of Work in America
McKinsey Digital (2023)
Superagency in the Workplace: Empowering People to Unlock AI’s Full Potential at Work
McKinsey Digital (2023)
The Economic Potential of Generative AI: The Next Productivity Frontier
Task Automation KPI
Replace 70%+ of manual search interactions with AI-driven answers.
Backed by McKinsey Digital and ResearchGate findings on wasted search time.

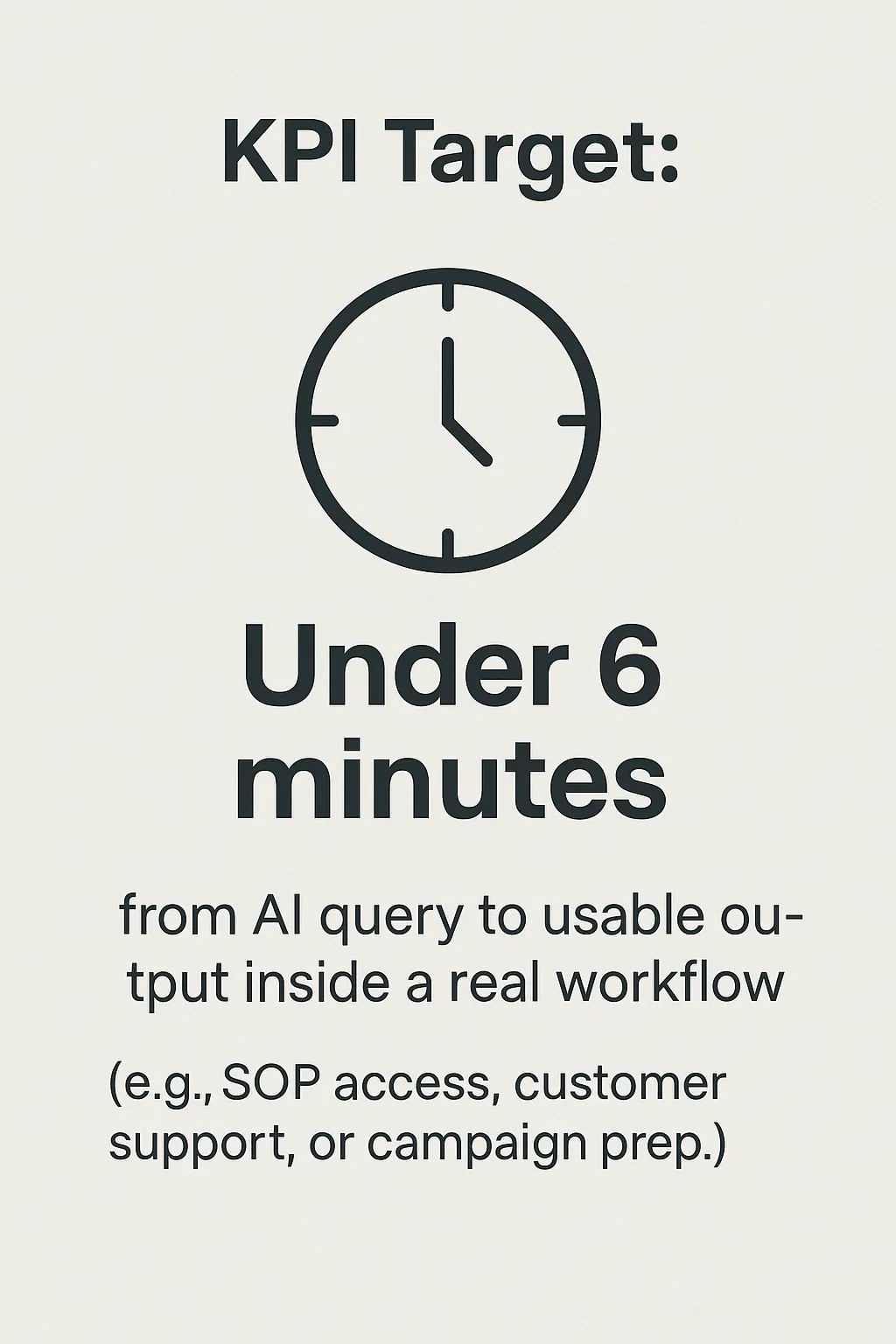
Time-to-Value
KPI Target: Under 6 minutes from AI query to usable output inside a real workflow
(e.g., SOP access, customer support, or campaign prep).
This reflects the shift from simply answering questions to delivering in-the-moment value—reducing the time spent navigating documents, folders, and disconnected systems.
Source: McKinsey Global Institute, A New Future of Work, 2024
A Challenge to Watch
If teams aren’t using it, find power users and let them lead. Highlight their wins (e.g., “Saved 2 hours this week on customer queries”) and share these examples with the entire team.
Days 61–90: Make AI Indispensable
Goal
AI should be viewed as business-critical.
AI Expansion Plan
- Fully automate high-friction, search-intensive workflows (e.g., automate customer query responses in support, report generation in finance).
- Expand AI to other teams (e.g., HR, finance, legal): Start with a pilot in a new department, using real wins to build momentum.
- Double down on real use cases that shorten deal cycles, reduce delays, or improve service quality (e.g., sales closing deals 10% faster with instant customer insights).

ROI Snapshot (Based on Modeling for a 500-Person Professional Services Company Australia)
- Baseline productivity loss: $2.9M/year
- Assumed AI impact (50% less time lost): $1.45M/year
- Estimated AI cost: $275K/year (based on New Dialogue AI’s per-user pricing for 500 users)
Net Annual Savings: $1.17M/year
- Scaled Impact: For a 100-person company, savings could be ~$234K/year; for a 1,000-person company, ~$2.34M/year.
Key KPIs
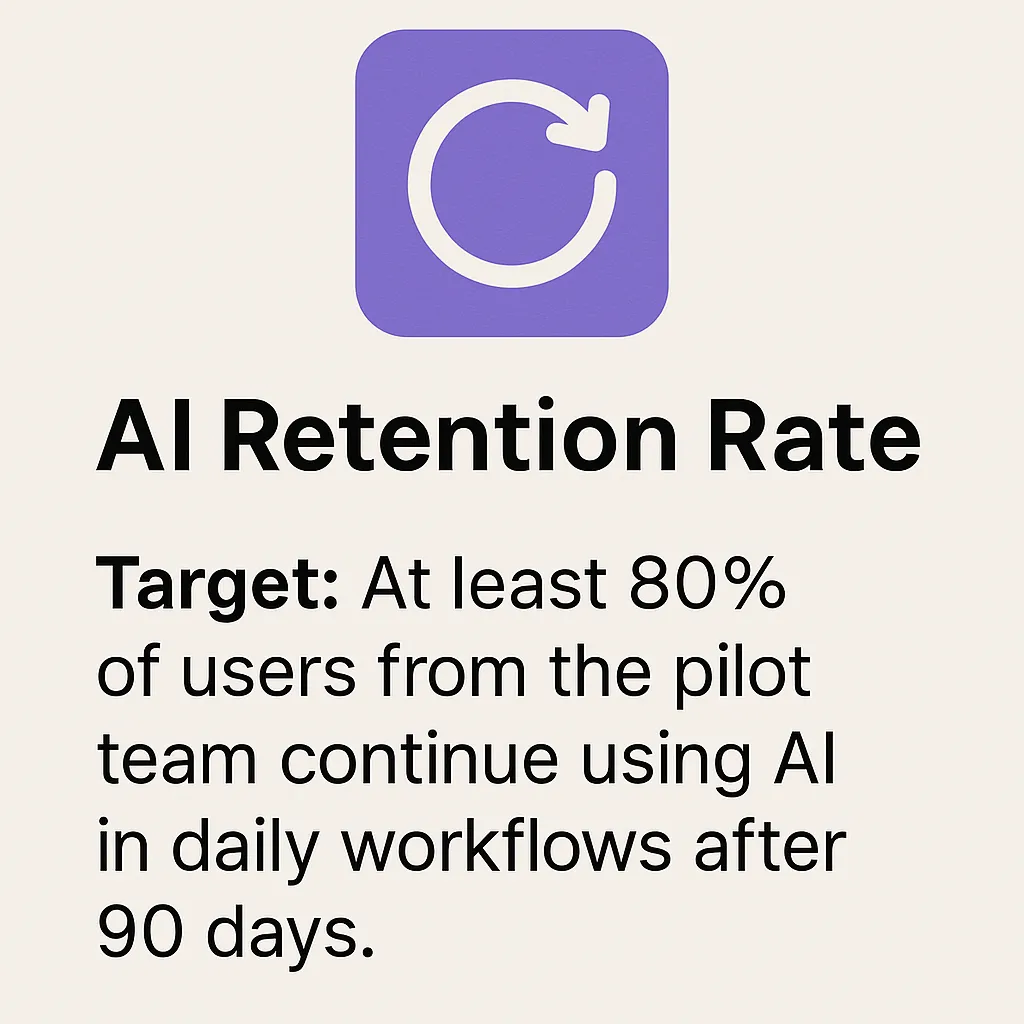
Retention Rate
Target at least 80% or more of users from the pilot team continue using AI in daily workflows after 90 days. This indicates AI is actually helping people do their jobs—not just being tested and forgotten.
Note: There is currently no industry-standard source that tracks AI usage retention rates within teams. This is a practical benchmark used by companies to assess real adoption and value.
Efficiency Gains
Aim for measurable improvements in how quickly and accurately work gets done. Well-designed AI workflows can improve task speed and output quality by up to 40%, especially in repetitive, search-heavy, or document-based work. Accenture, Technology Vision 2023

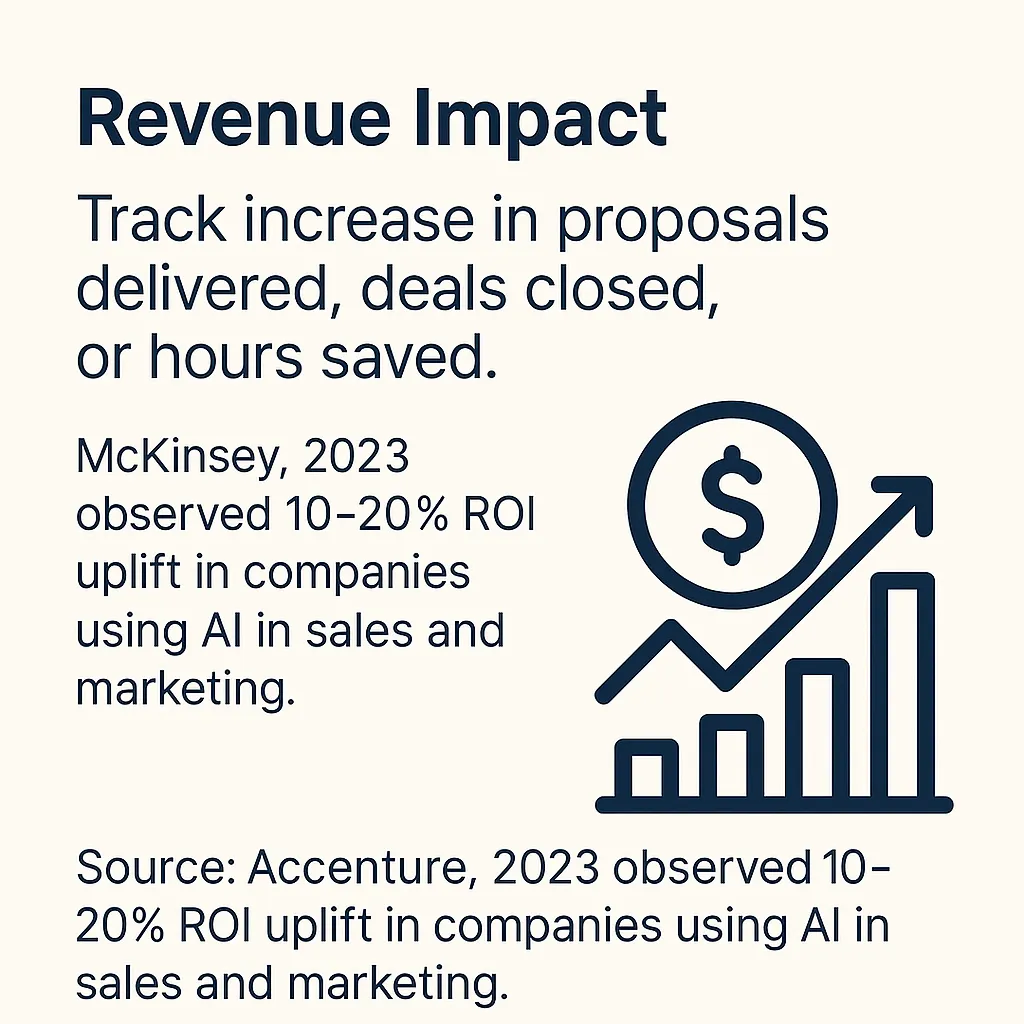
Revenue Impact
Track business outcomes like proposals delivered, deal velocity, or hours saved on high-value work. McKinsey estimates that generative AI can drive 5–15% productivity gains in marketing—translating to significant ROI uplift in revenue-focused functions.
This is a practical benchmark based on how fast successful AI rollouts tend to spread across cross-functional teams.
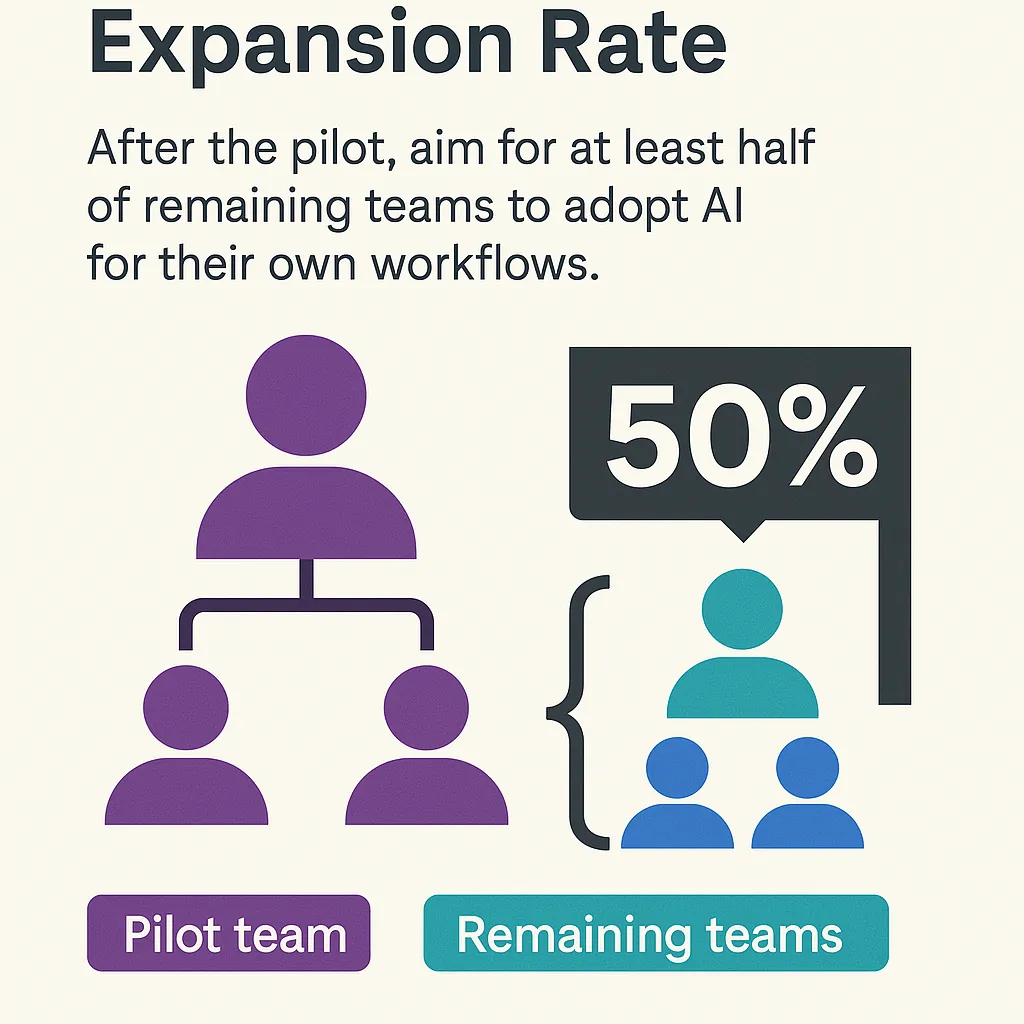
Exec Tip
If your team wouldn’t miss AI if it disappeared—you haven’t gone deep enough. Revisit Days 31–60 and co-design use cases with your people to solve their daily pain points.
Tracking AI Execution Weekly & Midpoint Checkpoints
Use these checkpoints to stay on track and ensure your AI rollout delivers measurable results.
Weekly Checkpoints
Is AI reducing response time in real workflows?
Are users actively engaging with AI, not just testing it?
Is AI generating useful, actionable outputs?
Are early time or cost savings starting to show?
Midpoint Review (Day 45)
By this stage, you should begin to see signs of adoption and value. If not:
Adoption Rate:
Target 50%+ active users engaging with AI.
If below, run targeted retraining or team-specific demos.Automation:
Target 30%+ of repeatable tasks supported or automated by AI.
If below, refine workflows or improve data structure.Realized Savings:
Check whether you’re on track to meet your projected annual savings
(e.g., based on AI’s impact on time, search reduction, or workflow efficiency).
If lagging, adjust AI scope, improve data quality, or expand integration points.
Industry Benchmarks to Guide Progress
What Comes Next
This playbook has laid out a clear, practical roadmap for AI implementation. Now it’s time to put it into action.
Leading organizations aren’t just planning for AI—they’re deploying it to automate workflows, retrieve information instantly, and streamline execution.
That’s exactly what New Dialogue AI is built for:
- Instantly find the right information with AI-powered retrieval
- Automatically prepare reports, summaries, or comparisons from your own data
- Build secure, chat-based tools for your teams or customers—no dev team required
How Organizations Move Forward with AI
Run a structured AI trial – Start with a focused use case and measure the impact
Refine your AI strategy – Adapt based on real results, not theory
Scale with the right platform – Choose AI that drives execution, not complexity
Ready to Get Started?
[Start a Free Trial] – Hands-on, no risk
[Book a Demo] – See how New Dialogue fits your workflows
[Talk to Us] – Let’s discuss AI execution in your business
AI execution isn’t about experimenting. It’s about making work faster, smarter, and more efficient.
Let’s get started.
About New Dialogue AI
New Dialogue AI is a platform developed by CentricMinds, an Australian Enterprise SaaS company working with enterprise customers across Asia-Pacific and North America.
Following our MVP development in 2023, New Dialogue AI has now been deployed to a range of enterprises (private, public and government) to enable team members to prompt their internal knowledge, privately and securely. New Dialogue AI enables users to generate AI powered chatbots targeting unique internal and external audiences, while securely accessing pre-defined content and data. This enables an organisation to empower their teams across all departments to chat with internal content, generate assets (proposals, reports, information graphics, and more) that is contextually relevant to their operational knowledge.
New Dialogue AI is available under our public SaaS offering. You can start a free 7-day trial here: https://www.newdialogue.com/free-trial
New Dialogue AI is also available to be deployed to private AWS and Azure tenancies under an enterprise subscription model.
Disclaimer
All productivity and revenue estimates are based on publicly available benchmarks and internal modeling by New Dialogue AI. The savings, productivity gains, and ROI projections in this 90-day plan are estimates based on modeling and industry benchmarks, including data from ResearchGate (2024), McKinsey Global Institute (2023–2024), Accenture (2024), and New Dialogue AI internal analysis. Actual results may vary depending on factors such as employee adoption rates, data quality, workflow complexity, and specific use case implementation. The baseline productivity loss (e.g., $2.9M/year or $2.3M/year) assumes an average search time of 25.7 minutes/day or 20 minutes/day per employee, respectively, which may differ across organizations. Costs are based on New Dialogue AI’s per-user pricing as of March 2025 and exclude potential additional expenses (e.g., training, integration). This plan is intended as a strategic guide, not a guaranteed outcome. Consult with New Dialogue AI for a tailored assessment.
References
MIT Sloan – How Generative AI Can Boost Highly Skilled Workers’ Productivity
New Dialogue – Internal use case testing across 600+ real employee queries
New Dialogue modelling based on ResearchGate (2024), ABS salary data, and IbisWorld industry revenue figures
Harmonic Security – New Research: The Data Leaking Into GenAI Tools, March 2024
Gartner – Lack of AI-Ready Data Puts AI Projects at Risk, February 26, 2025
Transform your knowledge into results
Experience how New Dialogue AI can transform your operational knowledge into
results that accelerate business outcomes.